Guide to Data Pipeline Design: Steps and Patterns
Data pipelines are the backbone of modern data-driven organizations. They’re the key to successfully gathering data and getting the most value from it. They help your organization improve data access to data, enable insightful business intelligence, and provide the scalability necessary for your growing data demands.
As the volume and complexity of data continue to increase, investing in robust data pipelines becomes not just an advantage but a necessity for organizations looking to get the most from their data as a strategic asset. In this article, you’ll find background on data pipelines and learn how you can strategically create one and implement it in your organization.
What is a data pipeline?
A data pipeline is a structured set of processes designed to automate the movement and transformation of data. Similar to how water pipelines transport water to cities where it can be filtered and used, data pipelines transport data from various sources to a destination where it can be analyzed and used for decision-making.
A data pipeline is designed to make collecting data easier, more efficient, and more consistent across different systems. A well-organized data pipeline empowers an enterprise to process large volumes of data, enabling insights and analytics that drive operational and strategic initiatives. By automating data workflows, pipelines eliminate manual data handling, reduce errors, and improve scalability in handling diverse data formats from multiple sources.
To fully understand what a data pipeline is, you’ll also need to understand how it’s different from data integration. While both data pipelines and data integration involve handling and consolidating data, they serve distinct roles.
A data pipeline is a broader term referring to the end-to-end system that automates data flow from source to destination with transformation, validation, and processing. Data integration, on the other hand, focuses on combining data from different sources into a unified format. It often uses extraction, transformation, and loading (ETL) tools to merge structured and unstructured data for consistent organization.
Essentially, data integration is a crucial part of a data pipeline, but a pipeline also encompasses additional functions such as automation, orchestration, and real-time processing to ensure seamless data movement across an organization’s ecosystem.
The importance of data pipeline design
Before beginning to build a data pipeline, you need to understand the importance of solid pipeline design. The way a data pipeline is designed can drastically affect its efficiency, playing a crucial role in the quality of your data, the speed and accuracy with which you can make executive-level decisions, and how well your pipeline can be scaled down the road.
Enhancing data quality and accessibility
One of the most significant reasons why good data pipeline design is important is its impact on data quality and accessibility. By automating the ETL of data from multiple sources, pipelines help eliminate inconsistencies, errors, and redundancies. They enforce standardization and validation, ensuring that only clean, reliable data is available for analysis.
Additionally, data pipelines improve accessibility by transferring data to centralized repositories such as data warehouses or data lakes, making it easier for teams across an organization to access the information they need without delays or manual intervention.
Enabling data-driven decision-making and business intelligence
Data pipelines are must-haves for competitive organizations, playing a fundamental role in decision-making and business intelligence. Companies rely on real-time and historical data to make strategic choices. Without automated pipelines, decision-makers would struggle with outdated or incomplete information, leading to inefficiencies and missed opportunities.
By continuously processing and delivering up-to-date data, pipelines enable analytics platforms, dashboards, and artificial intelligence models to function with high accuracy. This empowers businesses to identify trends, forecast outcomes, and respond proactively to market changes, ultimately leading to more informed and data-driven decisions.
Ensuring scalability and performance
Careful data pipeline design offers significant benefits in scalability and performance. As businesses grow and handle increasing volumes of data, manual or ad hoc data processing methods become impractical. Data pipelines provide a structured, automated approach that can scale with demand, ensuring that large data sets can be processed efficiently without bottlenecks.
They also optimize performance by distributing workloads, automating workflows, and integrating seamlessly with cloud-based architectures, which enhances flexibility and reliability. With well-designed data pipelines, organizations can efficiently handle massive amounts of data from diverse sources without compromising speed or accuracy.
Supporting real-time analytics and reducing IT burden
Scalability is particularly crucial for companies dealing with real-time analytics, machine learning applications, and large-scale customer interactions where data needs to be continuously processed and analyzed. Additionally, automated pipelines reduce the burden on IT teams by minimizing manual data management tasks, allowing them to focus on optimizing infrastructure and developing innovative solutions.
Components of a data pipeline
A well-structured data pipeline consists of several essential components and processes. Every data pipeline has essential pieces, like:
Data sources
Data sources are places where data is generated, such as databases, APIs, cloud storage, third-party services, or IoT devices. Your data can be structured, unstructured, or semi-structured.
A data ingestion component
Data ingestion happens when raw data is collected from sources and then fed into the data pipeline. Data can be ingested in batches or be streamed in real time.
Data processing and transformation capabilities
This step involves cleaning, filtering, aggregating, and formatting the data to ensure consistency and usability. It often includes ETL or extract, load, transform (ELT) processes. Transformation and processing make sure the data is clean, accurate, and in a usable business format.
Orchestration and workflow management
These components ensure that tasks run, whether in sequence or in parallel, to manage dependencies and scheduling execution. It’s also essential for managing resources so a sudden wave of data doesn’t overwhelm your network.
A data storage layer
A data pipeline has to have a storage layer where processed data is stored, such as in warehouses, lakes, or databases, making it accessible for analysis. Where and how you store the data will depend on what you want to use the data for and how easily you need to retrieve it.
Monitoring and error handling components
To keep data accurate and make sure it’s still moving, monitoring components track the pipeline’s performance, detecting failures or inconsistencies and triggering alerts or corrective actions when needed.
Common data pipeline design patterns
There’s no one right way to design a data pipeline. Your pipeline can reflect the unique setup of your organization, what you plan on doing with the data, and more. Here are some common data pipeline design patterns to get you started.
Batch processing
Batch processing is one of the most traditional data pipeline designs, where data is collected over a period and processed in large chunks at scheduled intervals. This approach is ideal for scenarios where real-time data isn’t critical, such as generating financial reports, processing payroll, or consolidating nightly sales data.
While batch processing may introduce some latency, it is highly efficient for handling large volumes of structured data with well-defined processing windows.
Real-time processing
Real-time processing (as opposed to batch processing) continuously ingests and processes data as it is generated. This enables immediate insights and actions. This design is essential for applications like fraud detection, stock market analysis, and IoT monitoring, where even milliseconds of delay can be costly. Real-time pipelines rely on technologies like Apache Kafka, Flink, or Spark Streaming to ensure low-latency data movement and analysis.
ETL
The ETL pipeline design follows a structured process where data is first extracted from multiple sources then transformed into a standardized format and loaded into a data warehouse or other storage systems. ETL is widely used in business intelligence and reporting, ensuring that raw data is cleaned and optimized before analysis.
While effective for structured data, ETL processes can become time-consuming as data volumes grow, leading to shifts toward alternative models like ELT.
ELT
ELT flips the traditional ETL sequence by first loading raw data into a storage system, such as a data lake, and then applying transformations as needed. This design leverages modern cloud computing and scalable storage solutions, allowing organizations to store massive data sets without upfront transformations.
ELT is particularly beneficial for big data analytics and machine learning because it provides flexibility in how and when data is processed.
Microservices architecture
Microservices architecture introduces a modular approach to data pipelines, breaking down processes into independent, loosely coupled services that communicate with each other via APIs. This design enhances scalability and flexibility, allowing teams to build, deploy, and manage individual components separately.
Microservices-based pipelines are ideal for large, distributed systems where different data processing tasks need to run independently while maintaining seamless integration across the organization.
Steps for designing a data pipeline
Designing an enterprise data pipeline requires a strategic approach to efficiently collect, process, and store extensive amounts of data. Here’s a step-by-step guide to designing a data pipeline that’s scalable and reliable.
1. Establish goals.
Before investing any time or money into a data pipeline, you should know exactly what your goals are to help guide your decisions through the rest of the pipeline-building process.
Ask yourself what metrics do you want to focus on? What do you want to do with your data? What kinds of resources can you dedicate to data processing?
2. Document core business processes.
When designing and implementing an enterprise data architecture pipeline, organizations need to remember that the architecture must support the core business processes and not the other way around. It’s vital to standardize and document core business processes across the entire enterprise. The better you document business processes, the more easily you’ll be able to build a pipeline that includes all necessary data and doesn’t take resources away from essential business functions.
3. Choose the right data ingestion methods.
Your data ingestion method will depend on how quickly you need the data and how much of your resources you want to spend on data processing. Enterprises typically ingest data from multiple sources, such as transactional databases, APIs, logs, IoT devices, and third-party platforms. Depending on the use case, data can be ingested in batch mode (collecting and processing data at scheduled intervals) or in real-time, when streaming technologies process data continuously.
4. Choose your transformation process.
The right transformation process for you will depend on what types of data you have, how quickly you need it processed, and what kind of data modeling you want to use. Traditional ETL processes transform data before loading it into a data warehouse, making it structured and analysis-ready. In contrast, ELT leverages scalable cloud storage to hold raw data before applying transformations on demand.
To perform large-scale transformation efficiently, consider utilizing technologies like Apache Spark, dbt (data build tool), and AWS Glue. These tools are designed to handle complex data manipulations and processing tasks. The significance of this step lies in improving data reliability and making it easier for business intelligence and machine learning applications to derive insights from structured and processed datasets.
5. Set up data storage.
Organizations often use a combination of data warehouses, data lakes, and hybrid storage architectures to accommodate diverse data needs. Data warehouses like Amazon Redshift, Google BigQuery, and Snowflake are optimized for structured, query-driven analytics, making them ideal for business intelligence and reporting.
Conversely, data lakes such as AWS S3, Azure Data Lake, and Google Cloud Storage offer flexibility by storing raw, semi-structured, and unstructured data at scale, supporting big data and machine learning workloads. A well-architected pipeline integrates both approaches, enabling structured querying while retaining the flexibility to process raw data when needed.
6. Implement monitoring and governance.
Now that the bulk of your pipeline is complete, it’s time to implement the pieces that will refine your pipeline and keep your data clean and safe. Implement monitoring to track pipeline performance and identify issues. We also recommend implementing procedures for updating, troubleshooting, and optimizing your pipeline.
You should also implement policies for data access, security, and privacy. Data governance is crucial for regulatory compliance as well as for your company’s own safety and data quality.
Data pipeline design best practices
Implementing best practices when designing your data pipeline can be the difference between smooth, reliable data and a pipeline that gives you issues for months to come.
Documentation and version control
A critical best practice is including thorough documentation and version control strategies, which provide clarity on the pipeline’s structure, transformations, and dependencies. Proper documentation details data sources, ingestion methods, transformation logic, and storage destinations, ensuring that teams can understand and troubleshoot the pipeline efficiently.
Version control allows teams to track changes, roll back updates, and maintain consistency across different environments. By implementing clear documentation and structured version control, organizations can reduce operational risks and facilitate collaboration among engineers, analysts, and stakeholders.
Scalability
Scalability is essential when creating a data pipeline. Data ingestion needs can change quickly. Before you get too far into building a data pipeline, be sure that your resources and workflows will be able to support large amounts of data processing without decreasing performance. To efficiently manage resources, you’ll also want your pipeline to scale back down quickly.
Monitoring and alerting for performance and reliability
Implementing monitoring and alerting mechanisms is crucial for pipeline performance, ensuring that data flows seamlessly without unexpected failures or bottlenecks. Real-time monitoring tools help track key performance indicators like data latency, throughput, and error rates.
Establishing automated alerting mechanisms allows teams to detect anomalies like missing data, pipeline failures, or performance degradation and take corrective action before issues escalate. Implementing logging frameworks also enhances observability, making it easier to debug failures and optimize processing times.
Continuous Improvement and Optimization for Efficiency
Continuous improvement and optimization techniques help maintain pipeline efficiency as data volumes and business needs evolve. Optimizing query performance, parallelizing workloads, and leveraging distributed computing frameworks can significantly enhance processing speed. By implementing data quality checks and schema validation, erroneous or incomplete data can be flagged early in the pipeline.
Adopting an iterative approach to pipeline development enables organizations to deploy updates seamlessly while maintaining system stability. Regularly reviewing and refining the pipeline based on performance insights ensures long-term scalability and efficiency, allowing businesses to maximize the value of their data infrastructure.
Optimizing data pipelines with Domo
Domo helps companies get the most value out of their data assets by helping enterprises design, build, and optimize data pipelines. No matter what kinds of data sources you have, we can help you bring your data into a pipeline so you can use it in a way that informs business decisions. To learn more about how Domo can help you amplify your data, contact us today.
Check out some related resources:
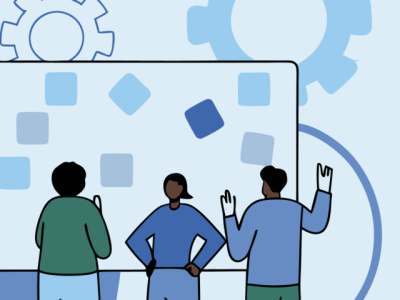
10 Best Data Visualization Tools for Marketers in 2025
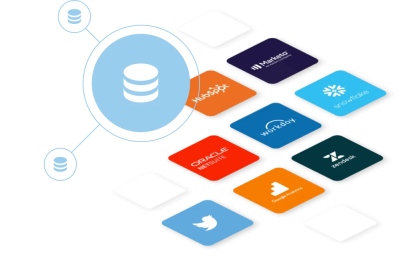
10 Best Data Integration Platforms in 2025
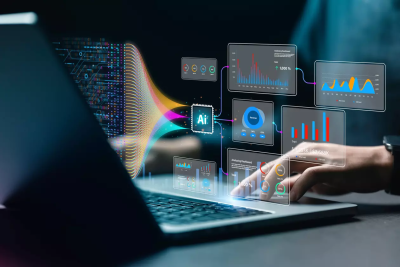