How modern BI & AI systems reduce human errors in data
It’s no secret that human error is common. After all, your employees are only human, and they’re prone to making mistakes. Data entry has an error rate as high as 4%. That means without verification, your company could be producing 400 errors for every 10,000 entries into databases.
There are numerous cases of human error when it comes to data management that should resonate with you and your team. If a NASA engineer uses inches instead of centimeters in a design, it could mean an explosion. If a doctor prescribes the wrong medication because it was notated by a human incorrectly, it could kill a patient. In data management, reducing error is critical to success and decision making. Data quality is next to sainthood as far as business intelligence is concerned.
Why does human error matter?
The cost of these errors can be quite high. They can be challenging to detect. They can lead to bias in your organization and its processes. You might not always have the time or resources to make the best decisions available. Humans aren’t always capable of being objective enough when it comes to data analysis, especially in highly emotional situations. Even if you think you’ve done everything right, you should still have processes in place and checklists for your team members. Humans can still make mistakes at any given point in time due to personal issues (stress from too much work), cognitive biases that lead them astray, or any number of other reasons.
What does this mean for businesses like yours? It means that without safeguards against human error, there’s a good chance that your company will end up making costly mistakes due to human error alone. That is outside of typical concerns and factors that affect business success, like market conditions.
In the United States alone, the potential cost of bad data caused by human error is roughly $3.1 trillion a year. That is money that could be spent on new product development, ensuring strong customer relationships, or expanding to new areas of business.
How does AI affect business intelligence?
In this day and age, when you collect so much data, your human intuition is not enough to process it. AI can help you discover trends in your data that might otherwise go unnoticed or be forgotten by the time your BI system gets them.
A modern business intelligence platform with integrated AI will help you automatically find patterns and trends in your data. You can also program it to recommend certain actions based on those insights. For instance, if the system discovers a drop in sales for a particular product, it can send an alert to the product manager responsible for that SKU so they’d know about it before next quarter’s results are available.
AI does not have bad days. There is no worrying about someone spilling coffee on an AI’s freshly laundered suit on the way to work. It performs at optimal speeds and functions all hours of the day regardless of what is happening to your human assets.
What types of human errors occur in data analytics?
In data collection, the most common human error is collecting improper or insufficient data. For example, if you’re trying to collect data on a specific product line as part of a sales report, but an employee forgot to include that information in their weekly email, that missing information can throw off your analysis.
In data analysis, the most common human error is using incorrect calculations and formulas. For example, when analyzing your company’s sales reports, you may have accidentally used the average instead of the median in your calculations, which can skew your findings.
In data reporting, the most common human error is presenting inaccurate or incomplete information. For example, if you’re reporting on customer satisfaction in response to an email campaign and forget to include feedback from a few respondents on one side of the spectrum (either very positive or very negative), this could paint a skewed picture of customer sentiment overall.
In data storage, the most common human error is storing redundant files and failing to delete outdated documents. This makes it difficult for teams to find what they need and increases security risk since old files may contain sensitive information about customers or employees.
How can AI reduce human error?
Humans can misjudge the effectiveness of their decisions due to a lack of access to relevant data. But what if you have all the right data? Could AI still help?
In theory, making good decisions is a straightforward exercise. You need only ask yourself two questions:
- What is the goal I’m trying to achieve?
- How will my decision affect achieving that goal?
However, in reality, humans are not always completely rational and logical. This can lead to decision-making that is based on intuition rather than facts or evidence. As a result, supported by these intuitions, our initial preferences and biases may lead us to make bad choices.
AI can help you compare alternative options for achieving your goals more objectively and reduce human errors in decision-making by helping us understand how our decisions will affect our goals.
Specifically, this could help reduce errors like:
Confirmation bias: AI removes those revelations that match any preconceived notions or ideas from your team. This is helpful in BI because it takes the predictions we may have about a challenge or insight and sticks to only the data interpretation.
Speed at data crossover: AI is built to correlate information. Where a human may find it challenging to go from one data silo to another, an AI can make the lead easily and uncover previously unrelated information that is now relevant.
Honest reporting: An AI does not care if the report it generates shines a bad light on a process, team, or project. A human may change data to make a friend in accounting look good, even when they have made mistakes.
Accurate predicting: AI is not trying to sell you anything. There is no need to pump up sales figures or boost incorrect data based on hopeful outcomes.
Speed & big data: Even a small mom-and-pop shop is going to have mountains of data from online buyers and social media marketing. A human cannot keep up with these demands where an AI is built to handle multiple sources of information with endless volumes of data.
Why does data quality matter in BI?
It’s easy to overlook data quality when it comes to big data, especially when you’re inundated with so many numbers. But having a consistent and accurate set of information is key in the decision-making process. Think about it: if your data is misleading or your insights aren’t based on the most up-to-date info, how can you make sound business decisions? Quality data goes hand in hand with trustworthy insights. Consider these ways that poor quality can lead to negative impacts for organizations.
- Business decisions based on outdated, incomplete, or inaccurate information could easily result in revenue losses
- Poor customer experiences can decrease loyalty and drive customers away from the business
- The costs associated with poor data quality are often greater than fixing it
- Data quality lowers operational costs because your team spends less time fixing errors and more time on critical needs
- You can predict what will happen in your niche and with your competitors if you understand what the data is saying
- Marketing is reliant on accurate data. You do not want to waste time or money on campaigns that target the wrong markets
Avoiding the ripple effect
The point is to elevate your BI capabilities with honest, accurate, reliable, and verified data sets. You want to expand your decision-making processes, and that requires as few errors as possible. Once a mistake is made, it can create a ripple effect through the rest of your organization, where everyone from the marketing team to leadership is basing their KPIs and motivation on bad information.
AI is not going to replace humans in an organization. It is going to be used the same way any other tool is used: by helping businesses create and succeed at their missions. Human intelligence is still needed because we are capable of making broad connections and insights that a computer is not.
It only makes sense to focus our efforts on systems that ensure data quality. Over time, your BI system should provide a clean dashboard that integrates all of your data sources into an easy-to-understand overview so you can make decisions about the future growth of your company and move on to more pressing matters. None of that will be possible without the assurance of high-quality data, and AI is a great way to solidify that goal.
Check out some related resources:
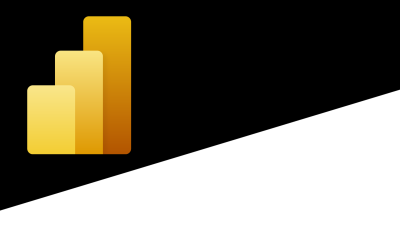
10 Power BI Alternatives and Competitors in 2025
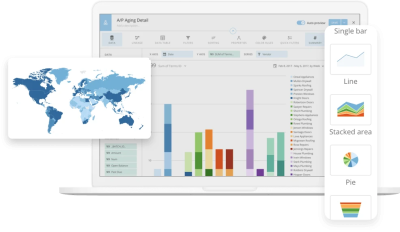
8 Best AI Tools for Data Visualization
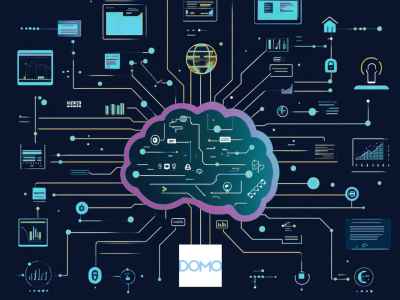