Streaming Data Pipelines: How To Build Them and Real-World Examples
Every moment, your systems generate data—from customer clicks and transactions to sensor readings and app events. But collecting that data isn’t enough. To stay competitive, you need to act on it as it happens.
That’s the value of a streaming data pipeline. Unlike batch processing, which analyzes data after the fact, streaming pipelines continuously capture, process, and deliver data in real time. They power a variety of applications, including live dashboards, personalized recommendations, instant fraud detection, and supply chain alerts.
In this article, we’ll break down what a streaming data pipeline is, how it works, key considerations for building one, and real-world examples across various industries. Whether you’re exploring use cases or ready to build, this guide will help you understand how to turn live data into immediate, impactful decisions.
What is a data pipeline?
A data pipeline is a series of processes that automatically move data from one system to another, transforming it along the way to prepare it for analysis or operational use. It connects data sources—like databases, applications, or APIs—to destinations such as data warehouses or dashboards. By automating data flow, pipelines ensure that information is consistently accurate, up to date, and ready for informed decision-making.
By understanding this fundamental definition of data pipelines, it will help clarify the function of a streaming data pipeline.
What is a streaming data pipeline?
A streaming data pipeline is a type of data pipeline designed to process data continuously and in real time as it’s generated. Instead of waiting to collect and analyze data in large batches, a streaming pipeline ingests, transforms, and delivers data on the fly—helping you make timely, informed decisions when every second counts.
How a streaming data pipeline works
As data is generated from sources, a streaming data pipeline ingests, cleans, and transforms it for downstream use. Here are the key steps involved in this process:
- Data sources: These are systems where data originates—like IoT sensors, clickstreams, social media feeds, or transactional databases.
- Ingestion layer: Streaming platforms capture and ingest raw data in real time.
- Stream processing: Once ingested, the data undergoes the extract, transform, and load (ETL) process to filter, aggregate, or enrich it. Stream processing frameworks remove unusable data and prepare data for real-time monitoring or analysis.
- Storage: Processed data is then stored in data warehouses, data lakes, or other storage solutions optimized for analytical queries.
- Analysis and action: The final component involves analyzing the processed data to derive insights, trigger alerts, or feed into machine learning models for predictive analytics.
Streaming vs. batch data processing
The main difference between streaming data processing and batch processing is timing. Batch processing collects data over a period of time, processes it as a group, and is ideal for historical analysis. It’s reliable but slower and often used for less time-sensitive data.
In contrast, streaming pipelines process data instantly. They’re built for agility and are ideal when quick insights are critical, such as fraud detection, system monitoring, or personalized digital experiences.
Here are a few other key differences:
Batch processing | Streaming processing | |
Data ingestion | Processes large volumes of data at scheduled intervals | Ingests and processes data continuously, in real time |
Latency | High latency; results are available after processing completes | Low latency; near-instant insights |
Monitoring requirements | Require less monitoring | Require continuous monitoring to ensure data integrity and handle errors |
Complexity | Simpler to implement and manage | More complex due to the need for real-time infrastructure |
Data storage | Data is stored first, then processed | Data is processed as it arrives, sometimes before full storage |
Resource efficiency | Efficient for large, periodic jobs | Requires always-on infrastructure, which can be more resource-heavy |
Use case | Historical reporting, trend analysis, ETL jobs | Real-time monitoring, fraud detection, personalized recommendations |
Benefits of a streaming data pipeline
The speed at which you can act on data is just as critical as the data itself. Streaming data pipelines empower you to harness real-time information, turning raw events into insights the moment they happen. Here’s why that matters:
Access data instantly
Traditional batch processing can delay access to key insights by hours or even days. Streaming pipelines deliver data as it’s generated, enabling you to respond instantly—whether that’s adjusting pricing, reacting to customer behavior, or spotting operational anomalies.
Real-time customer experiences
Modern customers expect personalization and responsiveness. Streaming data allows you to create tailored marketing campaigns, offer real-time recommendations, and detect issues like failed transactions or delivery delays before they escalate.
Operational efficiency at scale
Streaming pipelines reduce the manual work of data wrangling by automating the flow of data across systems, like from IoT sensors to CRM platforms. Teams get up-to-the-minute visibility into KPIs, inventory, supply chain shifts, or fraud risks without waiting for a batch job to run.
Improved forecasting and agility
When you can see what’s happening right now, you can better anticipate what comes next. Streaming analytics help teams spot trends early, run predictive models more frequently, and pivot strategies with confidence.
Foundation for AI and automation
Many AI and machine learning models thrive on real-time data. Streaming pipelines feed these systems with continuous, clean, and current inputs, fueling automated decisions that scale across marketing, finance, operations, and beyond.
In short, streaming data pipelines allow you to tap into what’s happening right now. For professionals who rely on timely, data-driven insights, this isn’t just a technical upgrade—it’s a strategic advantage.
Building a streaming data pipeline step-by-step
Building a data streaming pipeline might sound complex, but it’s about designing a system that captures, processes, and delivers data as it happens. Whether monitoring customer behavior in real time or powering live dashboards, a well-built pipeline gives you the visibility and responsiveness needed to make timely, informed decisions. Here’s a step-by-step guide to help you get started:
1. Define your goals and use case
Before choosing tools or writing code, clarify what you’re trying to achieve. Are you tracking real-time purchases? Monitoring IoT sensor data? Powering a recommendation engine? Your use case will shape the pipeline’s design.
2. Identify your data sources
List the systems generating real-time data. These could include:
- Application logs
- Web or mobile activity
- CRM or POS systems
- IoT devices or sensor networks
- External APIs or third-party services
3. Set up real-time data ingestion
You’ll need a way to capture and stream data from your sources as events occur. This layer acts as the gateway, collecting data continuously and moving it into your pipeline with minimal delay. Look for solutions that support high-throughput, fault tolerance, and scalability—especially if you expect large volumes of data or need to maintain reliability during traffic spikes.
4. Implement real-time data processing
Once data is ingested, it needs to be processed as it flows through the pipeline. This step involves transforming raw data into a more useful format—filtering out noise, combining data sets, enriching records, or running real-time calculations. Choose a processing approach that fits your needs for speed, scalability, and complexity, whether that’s applying simple rules or performing advanced analytics on the fly.
5. Define a clear schema
To keep your pipeline reliable and your insights accurate, it’s important to use a consistent format for your data. Defining a clear schema, such as field names, data types, and expected formats, helps all parts of the pipeline understand and work with the data effectively. A standardized structure also makes it easier to integrate new sources and reduce errors. It also streamlines downstream analysis.
6. Route data to your destination
After processing, your data needs to be sent to the systems where it will be stored, analyzed, or acted on, including:
- Storage solutions like data lakes or data warehouses
- Analytics platforms and dashboards like Domo
- Operational systems or tools, such as triggering alerts or automated workflows
The goal is to make the data accessible to the right people and systems in real time so it can power insights, trigger actions, or integrate into other workflows without delay.
7. Monitor and optimize your pipeline
Use observability tools to monitor throughput, latency, and error rates. To maintain reliability, you’ll need to fine-tune performance, set up alerts for failures, and build in retry mechanisms.
With these steps in place, you’ll have a strong foundation for a streaming data pipeline that delivers real-time value across your organization. As your data needs grow, you can scale and evolve your pipeline to keep insights flowing and decisions on point—no matter how fast things move.
Real-world examples of streaming data pipelines
Streaming data pipelines power some of the most dynamic and mission-critical systems across industries. These pipelines enable real-time decision-making and trigger automated actions by processing data the moment it’s created. Streaming pipelines are essential for organizations that depend on timely, data-driven actions to stay responsive, efficient, and ahead of the competition. Here are a few standout use cases:
Cybersecurity
In cybersecurity, speed is everything. Streaming pipelines allow security teams to detect threats the moment they occur—whether it’s an unauthorized login, suspicious network activity, or a spike in failed access attempts.
By continuously analyzing log data from firewalls, intrusion detection systems, and web activity, organizations can trigger real-time alerts and isolate affected systems to respond to incidents before they escalate.
Ecommerce
Ecommerce businesses rely on streaming data to personalize the customer experience and optimize operations. Data pipelines can track consumer behavior in real time, such as clicks, product views, and cart additions, to deliver tailored recommendations or limited-time offers instantly.
On the backend, streaming analytics can monitor inventory levels, detect payment fraud, or adjust pricing based on demand spikes—all while a shopper is still on the site.
Banks and Investment Firms
Financial institutions use streaming pipelines in numerous operations, from monitoring transactions to assessing risks and informing split-second decisions. For example, banks can stream credit card transactions to detect fraud patterns as they happen, stopping unauthorized charges before they’re approved.
Investment firms use streaming market data to power algorithmic trading, adjusting portfolios based on real-time fluctuations in stock prices, interest rates, or global events. This capability allows them to quickly respond to market changes, improving their competitiveness and efficiency in managing investments.
Logistics
Streaming data pipelines help logistics teams track shipments, vehicles, and inventory in real time. GPS and sensor data can power live dashboards, allowing teams to reroute deliveries and optimize warehouse operations.
By connecting these data sources as events happen, logistics companies reduce delays, improve accuracy, and deliver a smoother, more responsive customer experience.
Essential elements for a resilient data streaming pipeline
Building a successful data streaming pipeline means more than just moving data quickly. It’s about designing a system that can grow with your needs and stay reliable under pressure. Most importantly, it has to deliver value at every stage of the data lifecycle.
Below are six key factors to keep in mind when building your own streaming pipeline:
- Scalability: As your data volumes grow, your pipeline needs to scale alongside it. Choose tools and architectures that can handle high-throughput data ingestion and processing without compromising performance. Look for distributed systems and horizontal scaling capabilities to support future growth.
- Reliability: In a real-time environment, reliability is critical. A single failure in your pipeline can mean lost data or delayed insights. Build in failover mechanisms, retries, and message durability to ensure that your data keeps flowing—even when things go wrong.
- Optimizing data processing: Processing speed directly affects how quickly you can act on insights. Optimize your transformation logic to minimize latency and resource use. Consider windowing strategies, filtering early in the stream, and simplifying operations where possible to reduce overhead.
- Storage requirements: Even in streaming pipelines, storage plays an essential role—whether for long-term archiving, historical analysis, or buffering data between stages. Understand your data retention needs and choose storage solutions that balance cost, accessibility, and performance.
- Security: Streaming pipelines often move sensitive data, so security and governance can’t be an afterthought. Use encryption in transit and at rest, implement fine-grained access controls, and document your data flows to support auditability and compliance.
- Monitoring and observability: Real-time pipelines need real-time visibility. Set up dashboards and alerts to track throughput, latency, and error rates. Observability helps your team catch issues early, tune performance, and maintain trust in your data.
Increase performance and growth with real-time insights
Streaming data pipelines turn your constant influx of data into continuous opportunity. By capturing, processing, and delivering information in real time, they empower you to detect anomalies instantly, personalize customer experiences on the fly, and keep a pulse on your operations minute by minute. When timing defines success, streaming pipelines help you move from reactive to proactive with precision.
If you’re ready to move beyond static reports and start making decisions powered by live data,Domo can help you build a streaming pipeline that keeps you connected, informed, and confidently ahead of what’s next.
Check out some related resources:
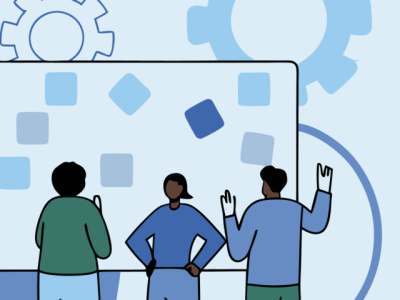
10 Best Data Visualization Tools for Marketers in 2025
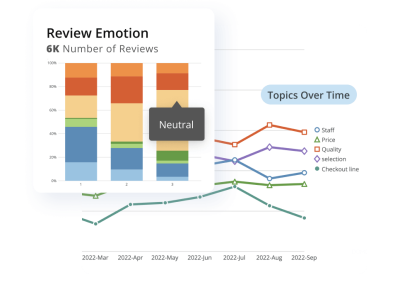
10 Best Business Intelligence (BI) Tools in 2025
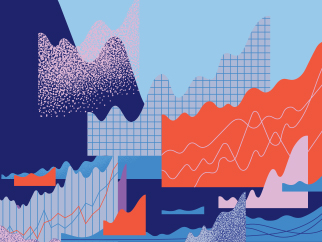