Guide to ETL Data Modeling: Process, Techniques, and Best Practices
ETL data modeling is the process of designing how data is extracted, transformed, and loaded into a database or data warehouse for analysis. It ensures data is structured in a way that supports efficient queries, reporting, and decision-making.
ETL data modeling can be a good way for your company to figure out the best way to structure your data based on your specific data needs. In this article, we’ll look at what ETL data modeling entails, some ways to use it to benefit your business, and share some best practices to help you set it up successfully.
What is ETL data modeling?
ETL data modeling is the foundation of an effective data strategy. It involves designing how data is extracted, transformed, and loaded (ETL) into a database or data warehouse to ensure it is structured for efficient queries, reporting, and business intelligence.
Without a solid ETL data model, organizations may struggle with inconsistent data, resulting in slow reporting times and incomplete insights. A well-structured model ensures data quality, accelerates decision-making, and allows businesses to scale as their data needs grow.
At its core, ETL data modeling consists of three key components:
- Extract: Data is pulled from any of multiple disparate sources, such as databases, spreadsheets, APIs, or cloud applications.
- Transform: The extracted raw data is cleaned, filtered, aggregated, and converted into a structured format.
- Load: The transformed data is stored in a data warehouse or database, making it readily available for analysis.
Unlike traditional data modeling, which primarily focuses on structuring databases for transactional systems, ETL data modeling is designed for analytics and reporting. It integrates data from a wide variety of sources, creating a unified data set for businesses to explore for deeper insights and make data-driven decisions.
Whether you’re working with sales figures, customer trends, or operational metrics, ETL data modeling ensures your information is organized, accurate, and ready to power meaningful analytics.
How does ETL data modeling work?
ETL data modeling follows a structured process to prepare data for meaningful analysis. Your company can use it to plan what data sources it’s going to integrate and how to use those data sources; it can then build a model for how and where that data will be stored to optimize current needs and future uses.
There are two processes to understand here: traditional ETL and ETL data modeling. Both processes follow similar paths and data modeling includes similar components to standard ETL. But ETL data modeling goes beyond traditional ETL by adding analysis into the early stages of your data processes.
An efficient data modeling process
The first step is extraction, where raw data is collected from various sources such as relational databases, CRM systems, web services, or even flat files. Because data often exists in different formats across multiple platforms, extraction ensures that all necessary information is gathered together into one place, ready for transformation. Your team will likely need some form of ETL tool to help manage this process.
Once extracted, the data moves into the transformation phase. This is where the raw information is cleaned, standardized, and structured. It involves:
- removing inconsistencies
- handling missing values
- applying business rules to ensure the data is accurate and usable
- aggregating data to summarize key insights
- enriching data sets with calculated fields
- normalizing or denormalizing information depending on reporting needs
This step is critical because poorly transformed data can lead to misleading analytics and unreliable business decisions.
Finally, the loading phase stores the transformed data into a data warehouse or database, making it accessible for business intelligence and reporting tools. A well-designed ETL data model ensures that this final data set is optimized for fast queries and seamless integration with dashboards and analytics platforms.
Understanding data modeling in ETL
On its own, data modeling is the practice of structuring and organizing data within a database to ensure it is stored efficiently and retrieved easily. It involves defining how data elements relate to each other, setting up schemas, and optimizing data storage for specific business use cases.
Data models often include entity-relationship diagrams (ERDs) that illustrate how data tables connect and interact. Effective data modeling ensures that data is accessible, consistent, and structured in a way that supports analysis and reporting.
When data modeling is combined with the ETL process, it takes on an even greater importance. ETL data modeling focuses on designing data structures that support the extraction, transformation, and loading of data efficiently. Instead of merely storing raw data, ETL data modeling organizes it into logical groupings, defining relationships between tables and ensuring that transformations produce meaningful, usable data sets.
A well-structured ETL data model accounts for:
- Data lineage: Tracking where data originates from and how it changes throughout the ETL process
- Schema design: Ensuring that the data warehouse follows a structured approach, such as a star schema, snowflake schema, or data vault (described in more detail below)
- Performance optimization: Indexing and partitioning data to improve query speed and reduce redundancy
- Business logic enforcement: Embedding rules and calculations within the transformation phase to ensure data integrity and consistency
By integrating ETL processes with data modeling best practices, businesses can create high-quality data sets that provide deeper insights, enhance decision-making, and ensure data remains accurate and reliable over time.
Benefits of ETL Data Modeling
A strong ETL data modeling strategy plays a vital role in effective data management, ensuring that data is structured, reliable, and scalable. By combining ETL processes with well-designed data models, businesses can improve the accessibility, quality, and usability of their data. Below are the key benefits of ETL data modeling:
Enhances data quality
Organizes and structures data logically for easier access and interpretation, establishes standardized formats and relationships between data to reduce inconsistencies, and improves data accuracy so analysts can work with reliable information
Increases operational efficiency
Streamlines the ETL process by minimizing unpredictability and manual interventions, optimizes data workflows to reduce processing times, and automates repetitive tasks to allow data teams to focus on strategic initiatives
Promotes scalability
Accommodates growing data volumes and expanding ecosystems, supports the integration of new data sources without significant modifications, and maintains performance consistency as data complexity increases
Improves awareness and data governance
Provides better visibility into data sources and security measures, supports robust data governance policies and regulatory compliance, and enhances understanding of data relationships for easier management and integrity maintenance
Optimizes data warehousing and reporting
Structures data to accelerate querying and reporting processes, implements entity integrity (data reliability within an entity) using primary keys to prevent duplication, and ensures referential integrity (data reliability between entities) using foreign keys to maintain accurate relationships between tables
By combining ETL processes with effective data modeling, businesses can establish a structured, efficient, and scalable data ecosystem. This ensures data is not only accurate and consistent but also optimized for business intelligence and analytics, ultimately driving better decision-making across the organization.
ETL Data Modeling Techniques
A well-designed ETL data model helps transform raw data into meaningful insights. Different techniques are used to optimize data storage, retrieval, and analysis. Here are the most widely used ETL data modeling techniques:
Dimensional modeling:
This approach focuses on structuring data for easy querying and reporting. It consists of:
- Fact tables that store quantitative data (e.g., sales, revenue, profit)
- Dimension tables that hold descriptive data (e.g., customer demographics, time, product details)
- Dimensional modeling commonly used in OLAP (Online Analytical Processing) systems to facilitate business intelligence
Star schema:
Star schema is a simple yet effective dimensional modeling technique where a central fact table connects to multiple dimension tables. It is widely used because it simplifies queries and speeds up data retrieval.
Snowflake schema:
This is an extension of the star schema where dimension tables are further normalized to reduce redundancy. While it improves data integrity, it can make queries more complex and slightly slower.
Data vault modeling:
A highly scalable approach that separates data into three categories and is useful for environments where data is frequently changing:
- Hubs (unique business entities like customers or products)
- Links (relationships between hubs, like transactions or purchases)
- Satellites (additional descriptive details like timestamps or metadata)
Pre-aggregation (materialization):
Instead of relying on complex joins, this technique pre-aggregates data into summary tables, reducing the computational cost of queries. This is particularly useful for performance optimization in large datasets.
Wide tables (denormalization):
Unlike traditional star schemas, modern data warehouses are shifting towards using wide tables with many columns. This reduces the need for joins and speeds up query performance.
Grain definition:
The grain of a table determines what a single row represents. Defining the correct grain ensures that data can be accurately aggregated and queried and helps maintain data consistency and usability. In a sales table, a single row could represent:
- One sale per customer per day
- One sale per transaction
- One sale per product per region
Best Practices for Effective ETL Data Modeling
To ensure effective ETL data modeling, organizations should follow these best practices:
Choose the right data model:
Different use cases require different models. Dimensional models work best for analytics, while normalized schemas are ideal for transaction-heavy environments.
Document the ETL process:
Maintain detailed documentation, including entity-relationship diagrams, transformation rules, and attribute definitions to facilitate understanding and troubleshooting.
Optimize performance with materialization:
Pre-aggregating data can improve query performance by reducing the need for expensive joins.
Define and maintain data grain:
Clearly define what each row in a dataset represents to avoid inconsistencies in analysis.
Partition data for efficiency:
Distribute large tables into smaller partitions to improve performance and scalability.
Implement continuous testing:
Conduct unit testing, integration testing, and end-to-end testing to ensure that data transformations are accurate and reliable.
Leverage automation:
Use machine learning and AI-driven algorithms to automate parts of the data modeling process, reducing manual effort and improving accuracy.
By implementing these best practices, businesses can ensure that their ETL data models are scalable, efficient, and well-suited for modern data analysis needs.
Using Domo for ETL Data Modeling
ETL data modeling works well to help companies maximize the value of their data. By following best practices and leveraging modern ETL tools like Domo, organizations can improve data quality, streamline reporting, and enhance decision-making. Whether you’re managing small data sets or massive data warehouses, investing in a solid ETL data modeling strategy will set your organization up for long-term success.
Domo’s cloud-based platform simplifies ETL data modeling with powerful tools like Magic ETL, a no-code, drag-and-drop interface for easy transformations, and Adrenaline DataFlows, which automate complex data processing. AI-Driven Insights further enhance decision-making by providing real-time analytics based on clean, structured data.
By integrating Domo into their ETL workflows, organizations can optimize data processes, improve efficiency, and gain valuable insights faster. A strong ETL data modeling strategy, supported by tools like Domo, helps businesses stay competitive, scalable, and data-driven in an increasingly complex landscape.
Ready to get started? Talk with Domo today.
Check out some related resources:
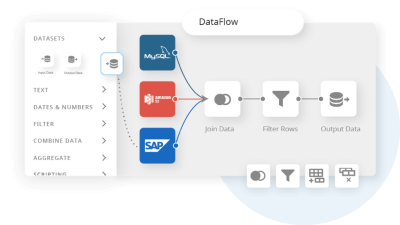
11 ETL tools to consider in 2025
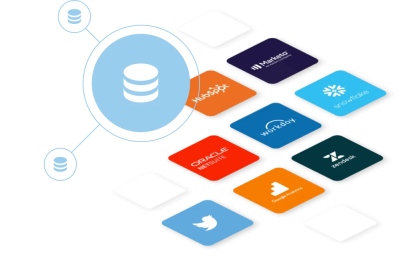
10 Best Data Integration Platforms in 2025
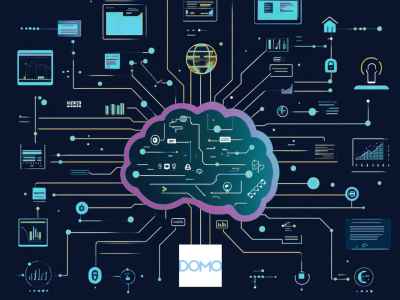