Data Analytics Strategy: What It Is and How To Create One
Every business owner knows that data is important. By understanding and analyzing the data your company produces, you can make better decisions that will help improve your bottom line.
In a world that is increasingly driven by big data, it is essential to have a data analytics strategy or framework in place. A strong data analytics strategy helps you make sense of the vast amount of data available to your business and use it to improve your decision-making.
So, what goes into building a data strategy? When considering data analytics, there are five essential elements you need to take into account:
- Collecting data
- Data analysis
- Reporting results
- Improving processes
- Building a data-driven culture
In this article, we will break down each of these five essential elements and discuss how they can help you successfully develop a data analytics strategy for your business.
What is data analytics?
Before we dive into the five elements of a data analytics strategy, it is important to first define what data analytics is.
Data analytics is the process of inspecting, cleansing, organizing, transforming, and modeling data with the goal of discovering useful information, informing conclusions, and supporting decision-making.
It uses tools and techniques to find trends and patterns, transforming raw data into actionable figures and reports that tell you where your business stands today. Data analytics also helps you determine where you want to be in the future and how you can achieve your goals.
You can’t take advantage of data analytics without first developing a data strategy. What is a data strategy? Simply put, it’s the framework that supports data analytics, defining how your company deals with data in all of its aspects, including:
- Data content
- Ownership of data
- Data quality
- How data is stored
- Data governance and security
Once that foundation is in place, your organization can develop a data analytics strategy to leverage data using specific people, processes, and technology. Your data analytics strategy must address your current business objectives, desired outcomes, and plan to implement them.
Business leaders will need to identify which employees and stakeholders to involve in the data analytics process, what tools they will use to analyze data, and how they will share information.
Why is a data analytics strategy important?
Your data analytics strategy is a long-term roadmap for how your organization will use data to solve problems and support your goals and growth. If you don’t know which objectives are most important to your business, what employees are responsible for data analysis, or how you will implement insights into actionable plans, you won’t achieve the results you’re looking for.
With strong data strategies, you can more easily realize the value of your data and achieve meaningful results. Your organization will be able to:
- Integrate your data and say goodbye to siloed information
- Have well-defined and consistent KPIs to measure your outcomes
- Make timely, data-informed decisions
- Explore historical data and forecast future metrics
- Reduce the time spent on manually collecting or preparing raw data
- Increase access and adoption of data throughout your company
- Improve data quality and accuracy
A well-developed data and analytics strategy benefits businesses in a number of ways, including:
Improving operational efficiency
Data analytics can help you identify inefficiencies in your business processes and workflows. Once these are identified, you can work to fix them, leading to improved efficiency and reduced costs.
Optimizing marketing campaigns
Data analytics can help you understand how customers interact with your brand and which marketing channels are most effective at driving conversions. By understanding this data, you can optimize your marketing campaigns for better results.
Identifying new business opportunities
Data analytics can help you spot trends in customer behavior and market demand. This information can be used to develop new products or services that meet the needs of your customers.
Improving customer service
Data analytics can help you identify areas where your customers are experiencing difficulty and identify solutions. This can help you provide a better customer service experience and improve customer loyalty.
The 7 elements of a data analytics strategy
Now that we have defined data analytics, let’s take a look at the essential elements of a data analytics strategy.
1. Align your data and business goals
The first stage of any data analytics process is to ensure your data strategy supports your overall business plans and objectives. This prevents you from wasting time or resources on the wrong data projects and helps you define and prioritize your data initiatives. Having an analytics strategy that supports your overall business goals can lead to greater success and better development of a data-driven workplace culture.
What steps do you need to take to align your goals? Here are several business and data strategy examples to consider:
- Identify areas of your business that would benefit from data analytics.
- Understand how specific tasks or activities contribute to business performance and goals.
- Learn what stakeholders and your leadership team want to accomplish, the questions they want answered, and what they want to measure.
- Discover how daily operations and workflows are currently run and how they could be improved.
- Determine which KPIs to track and compare your findings to industry averages or top competitors.
2. Appoint a data analytics team
Choose who will be responsible for conducting data analytics within your organization. Will you have one dedicated analyst or assign data responsibilities to different departments or teams? Defining clear roles and processes is essential. The operating model you select can depend on the size and needs of your business and include:
- Centralized operating model: offers greater structure where responsibilities lie under a specific role. It provides simpler data governance and more streamlined decision making.
- Decentralized operating model: analytics responsibilities are spread across departments or teams, like marketing, IT, accounting, and other business functions. This offers a more collaborative approach to data management and analysis.
- Hybrid model: a combination of the above two models, offering a centralized approach for more consistent data management and governance while allowing individual teams to be responsible for their own data initiatives.
You’ll also need to assess your analytics team’s skills, strengths, and weaknesses to see if your staff needs training, what tools would work best, and if you need additional technology or team members for things to run smoothly.
3. Collecting data
After aligning your goals and creating an analytics team, you then need to determine your data collection process. This involves gathering all of the data that your business produces, both internally and externally, associated with your analytics strategy. The data can come from a variety of sources, including:
- Operational systems: This includes data from systems such as ERP, CRM, and HRMS.
- Transaction data: This includes data collected from point-of-sale systems, e-commerce platforms, and financial databases.
- Web and social media data: This includes data from web analytics tools, social media platforms, and online surveys.
- Machine data: This includes data from sensors, RFID tags, and other connected devices.
Data collection can occur in a variety of ways, depending on the type of data and the source.
For example, operational data can be collected manually by extracting it from systems or automatically by using extract, transform, and load (ETL) tools.
Transaction data can be collected by downloading it from financial databases or automatically by integrating it with payment processing providers.
Web and social media data can be collected by setting up tracking scripts or using social media monitoring tools. And machine data can be collected by using sensors to track activity or by integrating with IoT platforms.
Once the data is collected, it needs to be cleansed and standardized so that it is ready for analysis. This process involves removing duplicate records, correcting errors, and formatting the data so that it can be easily analyzed.
4. Analyzing data
After the data is collected and cleansed, it is ready for analysis. Data analysis is the process of using statistical techniques to examine the data and extract useful information.
The goals of data analysis vary depending on the type of data and the business objectives. For example, data analysis can be used to:
Identify patterns and trends
Data analysis can help you identify patterns in customer behavior or market demand. This information can be used to make better decisions about products, pricing, and promotions.
Predict future outcomes
Data analysis can be used to build predictive models that can forecast future events. This information can be used to make decisions about inventory, staffing, and marketing.
Detect anomalies
Data analysis can help you identify unusual patterns that may indicate fraud or other problems. This information can be used to take corrective action to prevent losses.
Data analysis is typically done using data mining and statistical analysis software. These tools allow you to examine the data in different ways and extract useful information.
5. Reporting results
After the data has been analyzed, the results need to be reported. This step is important because it allows you to share your insights with others and make decisions based on the findings.
There are a variety of ways to report data analytics results, depending on the business and the audience. Some common methods include:
Presenting findings in a dashboard
A data dashboard is a graphical representation of the data that allows you to quickly see the key insights. Dashboards can be used to monitor performance over time or compare different data sets.
Generating reports
Reports are a written summary of the data that includes all the key findings. Reports can be used to share the results with others who may not be able to interpret the data themselves.
Creating infographics
Infographics are visual representations of the data that are designed to be easy to understand. Infographics can be used to communicate the results of data analytics to a wide audience.
The ultimate goal of reporting is to ensure that the data is being used to make better decisions. By sharing the results with others, you can ensure that the data analytics is having a positive impact on the business.
6. Improving processes
Data analytics is an ongoing process, not a one-time event. It may take some time to see results from the data analytics strategy. After you have collected and analyzed the data, you need to take action to improve the process.
Your data analytics strategy may need to be adjusted as new data is collected and new insights are gained. Be prepared to change the plan as needed. This step involves making changes to the way data is collected, processed, and analyzed. It may also involve changing the way decisions are made based on the data.
Data analytics is not a “set it and forget it” type of strategy. It requires constant attention and effort. Don’t give up if the data doesn’t immediately produce results. By constantly improving the process, you can ensure that data analytics is having a positive impact on the business.
7. Building a data-driven culture
Data analytics is not just about the data. It’s also about the people who use the data to make decisions.
To be truly data-driven, you need to build a culture in which everyone uses data to make decisions. This includes training employees on how to use data analytics and giving them access to the tools they need.
It also involves creating a culture of accountability in which everyone is responsible for using data to make better decisions.
Here are some tips on how to build a data-driven culture:
- Make data accessible
Give employees access to the data they need to make decisions. This includes making the data available in a format that is easy to understand — such as visualizations or reports.
- Train employees on how to use data
Teach employees how to use data analytics tools and how to interpret the data. This will help them make better decisions based on the data. Empower them to use data to solve problems.
- Encourage a data-driven mindset
Encourage employees to think about how data can be used to improve the business. This includes asking them to identify problems that can be solved with data and suggesting ways to use data to improve processes and solve problems.
- Create a culture of accountability
Hold employees accountable for using data to make better decisions. This will help ensure that data analytics is having a positive impact on the business.
Turning data analytics into action
For businesses that want to improve their bottom line, data analytics is essential. By understanding and analyzing data, businesses can make better decisions that will help improve their bottom line. With the right data analytics strategy, you can turn data into actionable insights that will help your business succeed.
RELATED RESOURCES
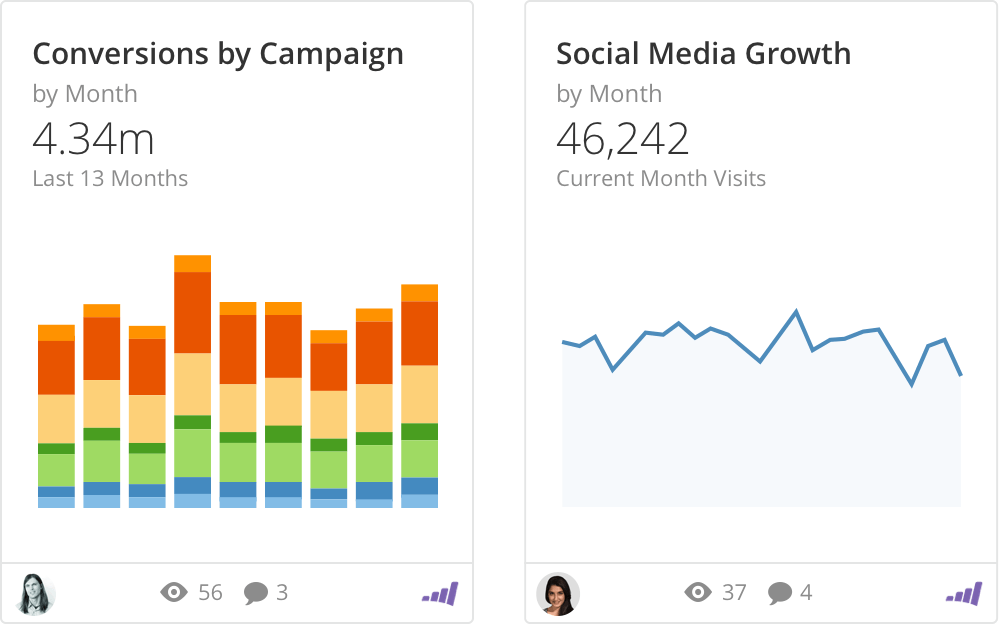
Article
10 Best Cloud Analytics Platforms in 2025
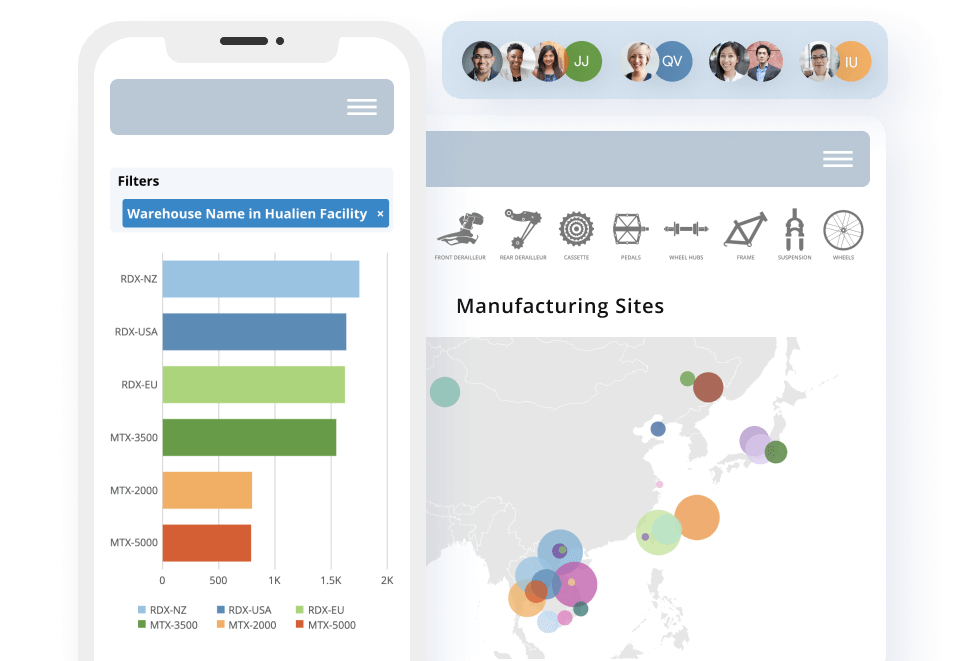
Article
8 SQL Visualization Tools to Consider in 2025
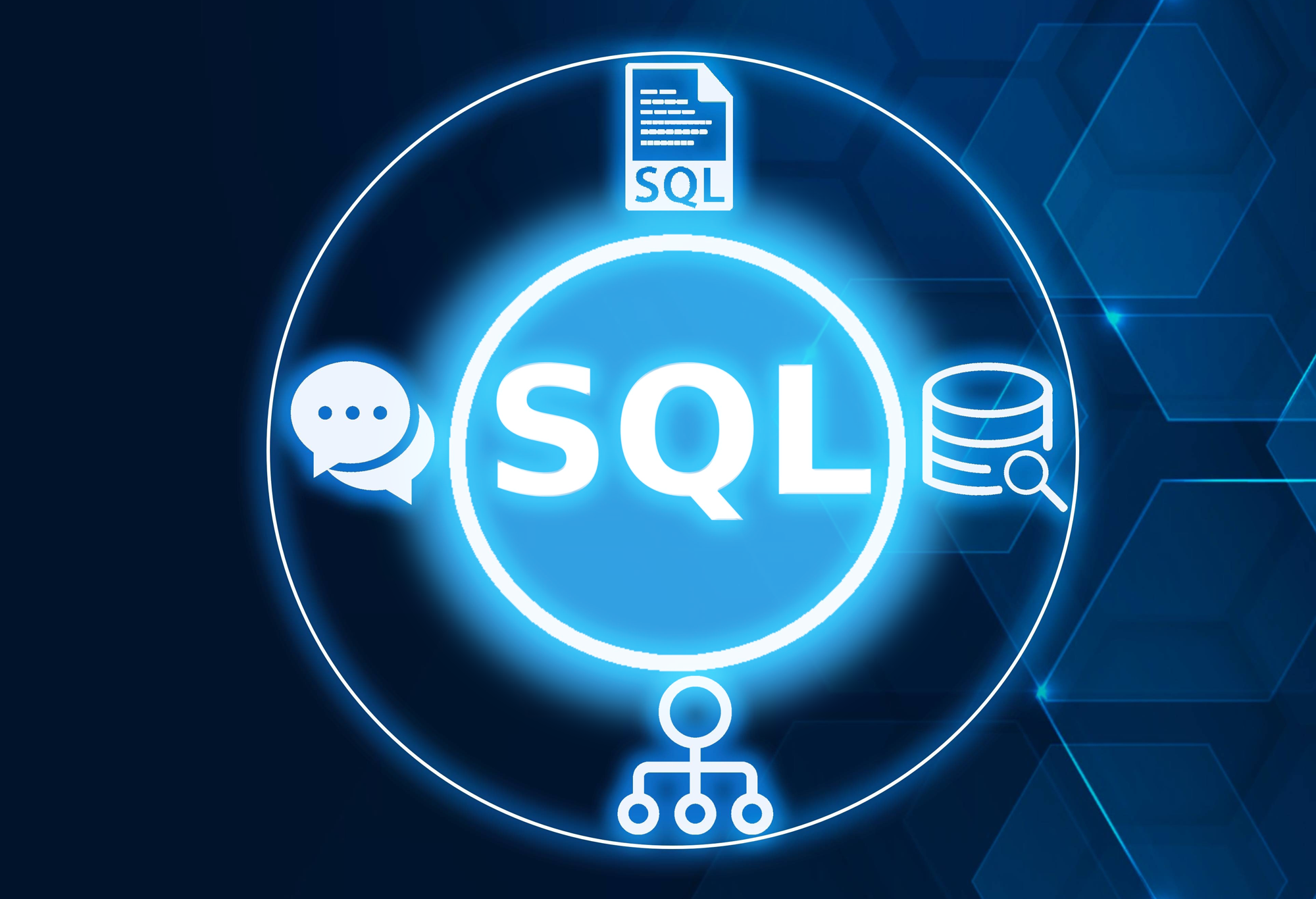
Article