Big Data and Artificial Intelligence: How They Work Together
Businesses have always relied on data to make smarter decisions, but with increasing technological advancements, almost every action businesses and customers make leaves a digital footprint. The vast amount of data available shows no signs of slowing down, and the volume and structure of this data are now too complex for humans to process and analyze through existing software and databases.
Fortunately, advances in technology also led to increased development of artificial intelligence (AI) and AI-powered tools, which offer the capabilities needed for big data analysis. In fact, AI and big data have an interdependent relationship, each relying on the other to operate effectively. By understanding the relationship between big data and AI, your business can take advantage of AI tools to gain real-time insights and have better outcomes.
What is Big Data?
Big data is the term for larger, more complex data sets that are too challenging for database tools to capture, manage, and analyze. It includes data from many sources and activities, including:
- Customer engagement
- Inventory levels
- Sales transactions
- Marketing campaigns
- Employee information
- Images and videos
- Daily business operations
- Web, app, and social media activity
Once you access and understand it, big data is extremely valuable to businesses, as you can make data-driven decisions that increase efficiency and revenue. However, analyzing and drawing insights from big data presents its own challenges. Big data arrives at an increasing volume and velocity, containing both structured and unstructured data—factors making it too difficult for traditional data processing tools to handle. This is where AI technology comes in, making analytics tools powerful enough to manage the growing influx of big data.
What is AI?
Artificial intelligence (AI) represents the computer science technologies that allow machines to perform tasks and simulate human capabilities. AI-based algorithms, natural language processing (NLP), and machine learning (ML) techniques are modeled on people’s decision-making processes, enabling computers to learn from data and make reasonable conclusions and predictions. The adoption of AI into business operations has grown dramatically, with 72% of global organizations reporting using AI in at least one business function.
What is AI for Big Data?
AI for big data is the merger of AI technologies with data analytics. AI-powered analytics, also known as augmented analytics, uses machine learning and AI algorithms to enhance the entire analytics process. AI can analyze and interpret all types of big data to derive accurate, meaningful insights quickly.
How does AI in big data accomplish this? AI models and algorithms are trained using datasets to recognize patterns and anomalies in the data and continuously learn and improve with use. AI can identify trends and correlations and make predictions based on data that would otherwise be too time-consuming or difficult for people to handle. Being able to transform raw data into strategic decisions quickly using AI gives businesses a huge advantage over their competitors.
The Relationship Between AI and Big Data
Artificial intelligence and big data are interdependent, relying on one another to operate. AI requires massive amounts of data to learn and refine its techniques for improved decision-making, while big data relies on AI-powered tools for timely, accurate analysis. The synergy between AI and big data is illustrated in the following processes:
Collecting Data
Big data is made up of enormous amounts of structured (numbers, dates, or structured text) and unstructured (images, audio, video, and large, text-based documents) data. Structured data can be modeled in tables and easily formatted for data storage and processing, while unstructured data are more complicated to collect and organize.
Both types of data originate from a variety of sources, including customer interactions, marketing and sales efforts, social media channels, website visitors, internet-connected devices and sensors, and so on. This data is the cornerstone of AI tools, allowing them to process and learn from the information. In turn, AI applications make it easier to collect and organize all types of big data for analysis.
Storing And Processing Data
AI algorithms and models require ongoing access to large datasets for training. The more data AI has access to, the better, as smaller amounts of data and low-quality data impact the accuracy and quality of its findings.
Big data storage technologies, like cloud-based data warehouses and data lakes, make it possible to store and process the vast quantities of raw data needed for AI applications. Cloud-based storage capacity allows for faster data transfer and reduces bottlenecks of data inputs and outputs. Businesses also rely on big data’ssecurity, compliance, and governance features to define and manage what AI can access for training.
Cleaning, Transforming, and Structuring Data
Big data brings information together from numerous different sources, platforms, and applications, but it has to be pre-processed before AI can analyze it. This process involves cleaning and transforming data into a structure suitable for use in models and algorithms.
Modern extract, transform, and load (ETL) technology is cloud-based. It takes raw data from its original source, transforms it into a format usable for AI tools, and loads the data into a targeted database for AI purposes. At the same time, AI technology, such as NLP and ML, isbeing incorporated into the ETL process for increased efficiency.
AI Modeling
After data is processed, it can be used in machine learning models. These models apply specific algorithms on big data inputs to make decisions or find programmed outcomes like pattern recognition or predictions without human intervention. However, some human supervision is needed to assess the validity of predictions and for reinforcement learning.
Training Models
AI and ML models aren’t useful without proper training, which is where big data comes in. Models rely on access to historical data to learn, using a specific algorithm to find patterns in data based on the target, or answer, you want the model to locate. Often, several algorithms are tested in models during training to find the best result. Once a model has been trained, it can find patterns and make predictions on new, unseen data in real-time.
Generating Real-time Insights
AI tools can reveal current trends, patterns, and anomalies in big data that could otherwise go unnoticed by human analysis. Businesses can use these intelligent insights to streamline their daily operations, enhance their services, adjust their product offerings, optimize their supply chain, reduce fraud or security risks, and more. AI’s data-informed predictions give companies even more advantages, as they can develop strategies based on sound information rather than gut feelings.
Innovating Research and Technology
Innovation in the fields of AI and big data go hand in hand. Big data heavily uses AI methods to operate and derive the most value, while AI is dependent on big data’s large volumes of information, cloud-based storage, and other tech like ETL tools to learn and refine its decision-making abilities. Research and innovation in one affects the other, making them more efficient and accurate.
Examples & Use Cases of AI for Big Data
Big data and AI play significant roles in data analytics and predictive analytics, using technology to automate and speed up the process. This decreases the time from data collection to insight, can also reduce errors within the process, and can result in cost savings.
Companies across all industries can benefit from AI and big data, as it empowers them to make informed decisions. Here are just a few of the many use cases of AI in big data:
- Financial Services: Financial institutions use AI to detect trends and anomalies in data, which can help them detect risks and fraud. They can also take advantage of AI-powered chatbots for customer service, ML models for credit risk assessment, and algorithm-based trading are also popular examples.
- Retail: AI tools can help predict consumer trends to optimize inventory and assist with product innovations. Many retailers also use AI-powered data to develop personalized recommendations for customers.
- Marketing: AI helps marketers better understand customer behavior and sentiment to segment audiences and deliver personalized marketing based on preferred methods, previous customer engagement, personal interests, or purchase history.
- Healthcare: AI can easily analyze patient charts, imaging, and other data to assist professionals in diagnoses. It can also develop personalized treatment options and predict patient outcomes.
- Manufacturing: Big data and AI enhance existing quality control systems to further improve product quality. It can also reduce equipment downtime by forecasting maintenance and equipment failures and predicting trends that will impact the supply chain.
- IT: IT professionals rely on AI in big data to monitor hardware and other tech infrastructure, predict maintenance needs, and identify cyber security risks to prevent attacks and downtime.
Are you interested in seeing how big data and AI can improve your business? Discover how Domo.AI combines AI innovations with our existing BI platform for powerful analysis and meaningful business insights.
RELATED RESOURCES
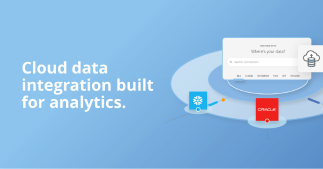
Article
4 ways to tell if your data is big data
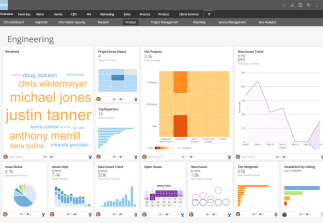
Article